Context in Machine Translation
Ingénierie et Architecture
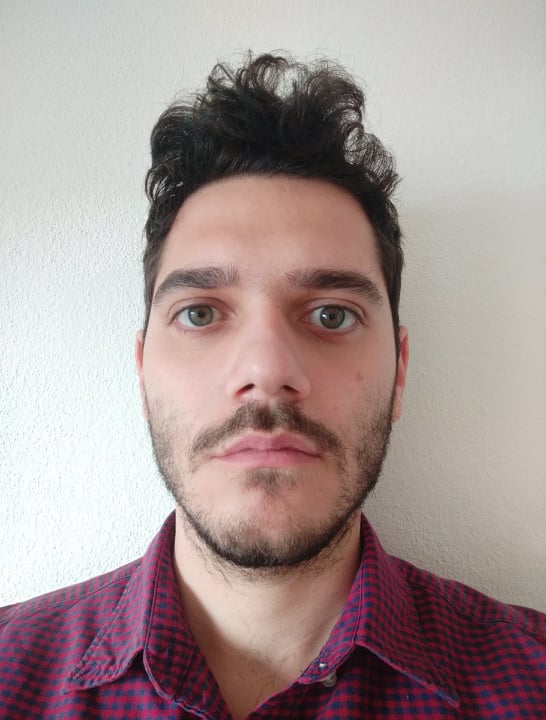
Machine Translation systems have achieved remarkable performance over the past few years. Part of the success of these systems lies in their ability to handle contextual dependencies at multiple scales. However, some parts of the current pipeline still do not have access to contextual information. We argue that the integration of context could bring further improvements to the quality of Machine Translation. At the scale of (sub)words, the use of contextual representations instead of surface form could improve the construction of bilingual vocabularies. At the scale of documents, the use of contextual information during the evaluation of Machine Translation systems can improve the accuracy of automatic metrics. In our work, we aim to explore the integration of contextual information in various scales and parts of the Machine Translation pipeline.