Context-Aware Monitoring of Hand Motor Primitives in Daily Life Activities: A Pathway to Objectively Assess Rehabilitation of Patients with Upper-Limb Neurological Impairment
Ingénierie et Architecture
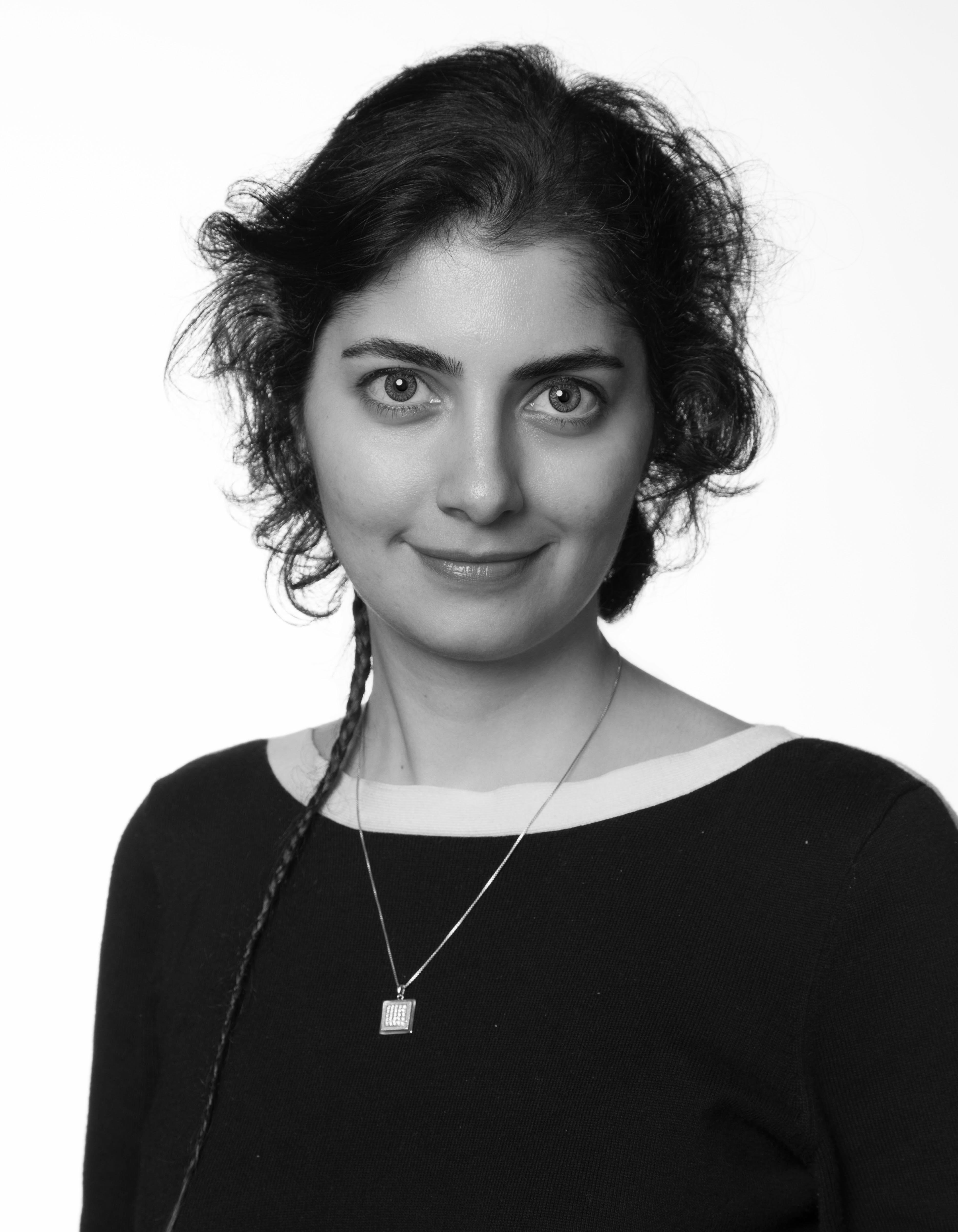
The hands, our silent performers in daily life, face overwhelming challenges when neurological impairments disrupt the simple tasks that compose our daily symphony. This thesis unveils a comprehensive framework for the objective monitoring of upper-limb rehabilitation, leveraging wearable sensors, an egocentric camera, and machine learning algorithms. It innovatively combines objective assessment tools with in-depth kinematic analysis to offer novel insights into hand motor primitive movement smoothness. This thesis focuses on granular motor movements, reaching, grasping, transporting and placing an object, since these motor primitive are building blocks of each simple and complex tasks.
Previous approaches to assessing upper-limb impairments have predominantly utilized multiple Inertial Measurement Units (IMUs), which can often compromise patient comfort during therapies or in a home environment. Alternatively, the marker-based stereophotogrammetry system, while accurate, demands substantial operational space and lacks portability and ease of use. Additionally, existing studies tend to focus on specific movements or, at most, one of the hand motor primitives. In contrast, this thesis explores four hand motor primitives, filling a notable gap in the literature. To the best of the author's knowledge, no existing framework combines IMUs on the wrists with egocentric vision on the shoulder to enhance the context in the objective assessment of upper-limb hand motor primitives. Moreover, patients with upper-limb neuromotor impairments often depend on standardized clinical scores, which are limited by operator dependence and susceptibility to ceiling and floor effects, failing to capture the full quality of upper-limb movements accurately. Consequently, this thesis aims to bridge the gap between subjective and objective assessments of upper-limb impairments, proposing a novel approach to enhance the accuracy and personalization of rehabilitation strategies.
An algorithm has been developed to log interactions of hand and object from an egocentric depth camera by utilizing foundational deep learning models. To make my framework wearable and more suitable for clinical practices, I introduced the application of a 2D egocentric camera enhanced by depth estimation. Subsequently, an algorithm has been deployed to obtain the quality of movement from IMU data for hand motor primitives. Finally, automatic video labeling for segmenting hand motor primitives using deep learning algorithms has been investigated.
Through these above mentioned series of milestones, the thesis validates the efficacy of the proposed system in real-world settings, emphasizing the importance of personalized, technology-assisted rehabilitation programs. Despite facing challenges such as data scarcity and the need for balanced patient datasets, the work lays a solid foundation for future advancements in the field, aiming to make rehabilitation more sensitive to subtle changes in motor recovery, accessible, accurate, and tailored to individual needs. Moreover, it complements traditional therapeutic strategies with technology-driven insights.
Overall, this thesis lays the groundwork for future research directions, including enabling clinicians to detect subtle changes in the smoothness of hand movements throughout the rehabilitation process, aiding in the design of more effective and personalized rehabilitation programs.
Keywords:
Context-awareness, Wearable Sensors, Digital Health, Machine Learning for Computer Vision, Upper-limb functional assessment, Inertial Sensors, Stroke Patients, Daily Life Activities